Fast computers, 5G networks and radar that passes through walls are bringing ‘X-ray vision’ closer to reality
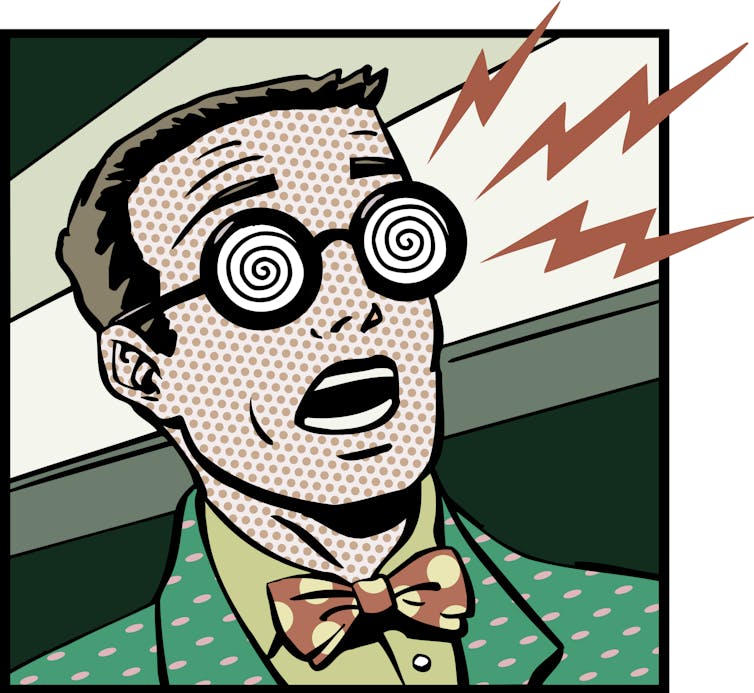
Within seconds after reaching a city, earthquakes can cause immense destruction: Houses crumble, high-rises turn to rubble, people and animals are buried in the debris.
In the immediate aftermath of such carnage, emergency personnel desperately search for any sign of life in what used to be a home or office. Often, however, they find that they were digging in the wrong pile of rubble, and precious time has passed.
Imagine if rescuers could see through the debris to spot survivors under the rubble, measure their vital signs and even generate images of the victims. This is rapidly becoming possible using see-through-wall radar technology. Early versions of the technology that indicate whether a person is present in a room have been in use for several years, and some can measure vital signs albeit under better conditions than through rubble.
I’m an electrical engineer who researches electromagnetic communication and imaging systems. I and others are using fast computers, new algorithms and radar transceivers that collect large amounts of data to enable something much closer to the X-ray vision of science fiction and comic books. This emerging technology will make it possible to determine how many occupants are present behind a wall or barrier, where they are, what items they might be carrying and, in policing or military uses, even what type of body armor they might be wearing.
These see-through-wall radars will also be able to track individuals’ movements, and heart and respiration rates. The technology could also be used to determine from a distance the entire layout of a building, down to the location of pipes and wires within the walls, and detect hidden weapons and booby traps.
See-through-wall technology has been under development since the Cold War as a way to replace drilling holes through walls for spying. There are a few commercial products on the market today, like Range-R radar, that are used by law enforcement officers to track motion behind walls.
How radar works
Radar stands for radio detection and ranging. Using radio waves, a radar sends a signal that travels at the speed of light. If the signal hits an object like a plane, for example, it is reflected back toward a receiver and an echo is seen in the radar’s screen after a certain time delay. This echo can then be used to estimate the location of the object.
In 1842, Christian Doppler, an Austrian physicist, described a phenomenon now known as the Doppler effect or Doppler shift, where the change in frequency of a signal is related to the speed and direction of the source of the signal. In Doppler’s original case, this was the light from a binary star system. This is similar to the changing pitch of a siren as an emergency vehicle speeds toward you, passes you and then moves away. Doppler radar uses this effect to compare the frequencies of the transmitted and reflected signals to determine the direction and speed of moving objects, like thunderstorms and speeding cars.
The Doppler effect can be used to detect tiny motions, including heartbeats and chest movement associated with breathing. In these examples, the Doppler radar sends a signal to a human body, and the reflected signal differs based on whether the person is inhaling or exhaling, or even based on the person’s heart rate. This allows the technology to accurately measure these vital signs.
How radar can go through walls
Like cellphones, radars use electromagnetic waves. When a wave hits solid walls like drywall or wood walls, a fraction of it is reflected off the surface. But the rest travels through the wall, especially at relatively low radio frequencies. The transmitted wave can be totally reflected back if it hits a metal object or even a human, because the human body’s high water content makes it highly reflective.
If the radar’s receiver is sensitive enough – a lot more sensitive than ordinary radar receivers – it can pick up the signals that are reflected back through the wall. Using well-established signal processing techniques, the reflections from static objects like walls and furniture can be filtered out, allowing the signal of interest – like a person’s location – to be isolated.
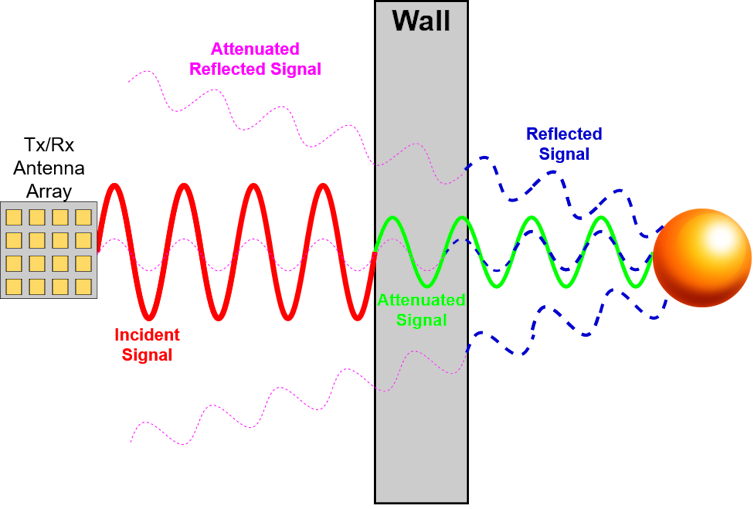
Turning data into images
Historically, radar technology has been limited in its ability to aid in disaster management and law enforcement because it hasn’t had sufficient computational power or speed to filter out background noise from complicated environments like foliage or rubble and produce live images.
Today, however, radar sensors can often collect and process large amounts of data – even in harsh environments – and generate high-resolution images of targets. By using sophisticated algorithms, they can display the data in near real-time. This requires fast computer processors to rapidly handle these large amounts of data, and wideband circuits that can rapidly transmit data to improve the images’ resolution.
Recent developments in millimeter wave wireless technology, from 5G to 5G+ and beyond, are likely to help further improve this technology, providing higher-resolution images through order-of-magnitude wider bandwidth. The wireless technology will also speed data processing times because it greatly reduces latency, the time between transmitting and receiving data.
My laboratory is developing fast methods to remotely characterize the electrical characteristics of walls, which help in calibrating the radar waves and optimize the antennas to make the waves more easily pass through the wall and essentially make the wall transparent to the waves. We are also developing the software and hardware system to carry out the radar systems’ big data analyses in near real-time.
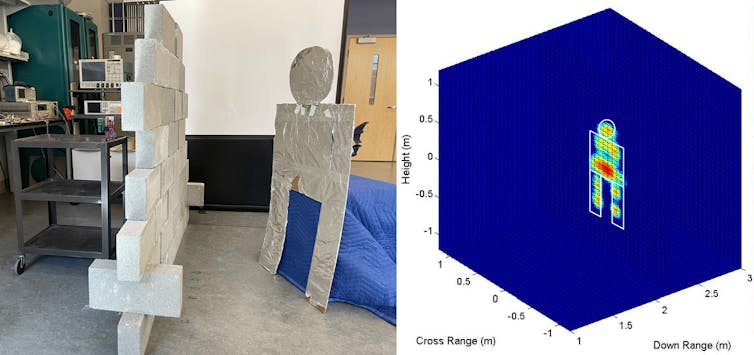
Better electronics promise portable radars
Radar systems at the low frequencies usually required to see through walls are bulky due to the large size of the antenna. The wavelength of electromagnetic signals corresponds to the size of the antenna. Scientists have been pushing see-through-wall radar technology to higher frequencies in order to build smaller and more portable systems.
In addition to providing a tool for emergency services, law enforcement and the military, the technology could also be used to monitor the elderly and read vital signs of patients with infectious diseases like COVID-19 from outside a hospital room.
One indication of see-through-wall radar’s potential is the U.S. Army’s interest. They’re looking for technology that can create three-dimensional maps of buildings and their occupants in almost real-time. They are even looking for see-through-wall radar that can create images of people’s faces that are accurate enough for facial recognition systems to identify the people behind the wall.
Whether or not researchers can develop see-through-wall radar that’s sensitive enough to distinguish people by their faces, the technology is likely to move well beyond blobs on a screen to give first responders something like superhuman powers.
[Understand new developments in science, health and technology, each week. Subscribe to The Conversation’s science newsletter.]
Aly Fathy, Professor of Electrical Engineering, University of Tennessee
This article is republished from The Conversation under a Creative Commons license. Read the original article.