EU law would require Big Tech to do more to combat child sexual abuse, but a key question remains: How?
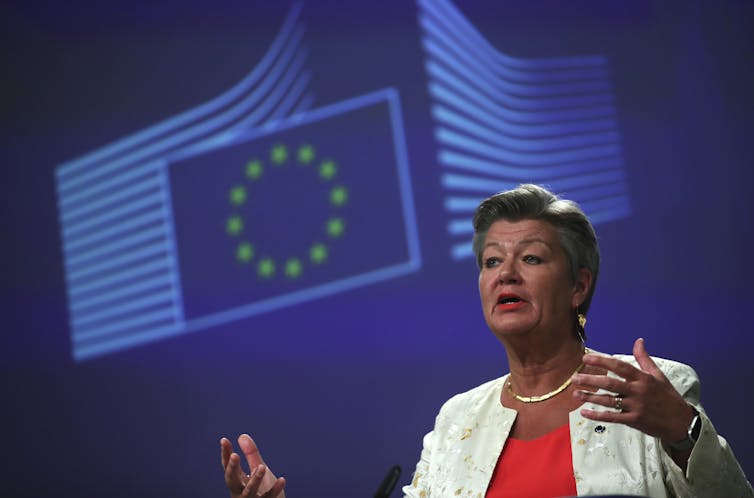
The European Commission recently proposed regulations to protect children by requiring tech companies to scan the content in their systems for child sexual abuse material. This is an extraordinarily wide-reaching and ambitious effort that would have broad implications beyond the European Union’s borders, including in the U.S.
Unfortunately, the proposed regulations are, for the most part, technologically unfeasible. To the extent that they could work, they require breaking end-to-end encryption, which would make it possible for the technology companies – and potentially the government and hackers – to see private communications.
The regulations, proposed on May 11, 2022, would impose several obligations on tech companies that host content and provide communication services, including social media platforms, texting services and direct messaging apps, to detect certain categories of images and text.
Under the proposal, these companies would be required to detect previously identified child sexual abuse material, new child sexual abuse material, and solicitations of children for sexual purposes. Companies would be required to report detected content to the EU Centre, a centralized coordinating entity that the proposed regulations would establish.
Each of these categories presents its own challenges, which combine to make the proposed regulations impossible to implement as a package. The trade-off between protecting children and protecting user privacy underscores how combating online child sexual abuse is a “wicked problem.” This puts technology companies in a difficult position: required to comply with regulations that serve a laudable goal but without the means to do so.
Digital fingerprints
Researchers have known how to detect previously identified child sexual abuse material for over a decade. This method, first developed by Microsoft, assigns a “hash value” – a sort of digital fingerprint – to an image, which can then be compared against a database of previously identified and hashed child sexual abuse material. In the U.S., the National Center for Missing and Exploited Children manages several databases of hash values, and some tech companies maintain their own hash sets.
The hash values for images uploaded or shared using a company’s services are compared with these databases to detect previously identified child sexual abuse material. This method has proved extremely accurate, reliable and fast, which is critical to making any technical solution scalable.
The problem is that many privacy advocates consider it incompatible with end-to-end encryption, which, strictly construed, means that only the sender and the intended recipient can view the content. Because the proposed EU regulations mandate that tech companies report any detected child sexual abuse material to the EU Centre, this would violate end-to-end encryption, thus forcing a trade-off between effective detection of the harmful material and user privacy.
Recognizing new harmful material
In the case of new content – that is, images and videos not included in hash databases – there is no such tried-and-true technical solution. Top engineers have been working on this issue, building and training AI tools that can accommodate large volumes of data. Google and child safety nongovernmental organization Thorn have both had some success using machine-learning classifiers to help companies identify potential new child sexual abuse material.
However, without independently verified data on the tools’ accuracy, it’s not possible to assess their utility. Even if the accuracy and speed are comparable with hash-matching technology, the mandatory reporting will again break end-to-end encryption.
New content also includes livestreams, but the proposed regulations seem to overlook the unique challenges this technology poses. Livestreaming technology became ubiquitous during the pandemic, and the production of child sexual abuse material from livestreamed content has dramatically increased.
More and more children are being enticed or coerced into livestreaming sexually explicit acts, which the viewer may record or screen-capture. Child safety organizations have noted that the production of “perceived first-person child sexual abuse material” – that is, child sexual abuse material of apparent selfies – has risen at exponential rates over the past few years. In addition, traffickers may livestream the sexual abuse of children for offenders who pay to watch.
The circumstances that lead to recorded and livestreamed child sexual abuse material are very different, but the technology is the same. And there is currently no technical solution that can detect the production of child sexual abuse material as it occurs. Tech safety company SafeToNet is developing a real-time detection tool, but it is not ready to launch.
Detecting solicitations
Detection of the third category, “solicitation language,” is also fraught. The tech industry has made dedicated efforts to pinpoint indicators necessary to identify solicitation and enticement language, but with mixed results. Microsoft spearheaded Project Artemis, which led to the development of the Anti-Grooming Tool. The tool is designed to detect enticement and solicitation of a child for sexual purposes.
As the proposed regulations point out, however, the accuracy of this tool is 88%. In 2020, popular messaging app WhatsApp delivered approximately 100 billion messages daily. If the tool identifies even 0.01% of the messages as “positive” for solicitation language, human reviewers would be tasked with reading 10 million messages every day to identify the 12% that are false positives, making the tool simply impractical.
As with all the above-mentioned detection methods, this, too, would break end-to-end encryption. But whereas the others may be limited to reviewing a hash value of an image, this tool requires access to all exchanged text.
No path
It’s possible that the European Commission is taking such an ambitious approach in hopes of spurring technical innovation that would lead to more accurate and reliable detection methods. However, without existing tools that can accomplish these mandates, the regulations are ineffective.
When there is a mandate to take action but no path to take, I believe the disconnect will simply leave the industry without the clear guidance and direction these regulations are intended to provide.
Laura Draper, Senior Project Director at the Tech, Law & Security Program, American University
This article is republished from The Conversation under a Creative Commons license. Read the original article.